Custom AI Development Services for HeathTech Applications
Unlock advanced healthcare solutions with our expertise in AI
Simbex’ experience with Artificial Intelligence (AI) began years ago with Predictive Algorithms which we used to develop training methods for minimizing risks of head-impact injuries.
Over time, our work in Algorithms, Machine Learning, Computer Vision, Deep Learning, and Analytics has allowed us to gain valuable AI experience across a broad variety of healthcare technologies.
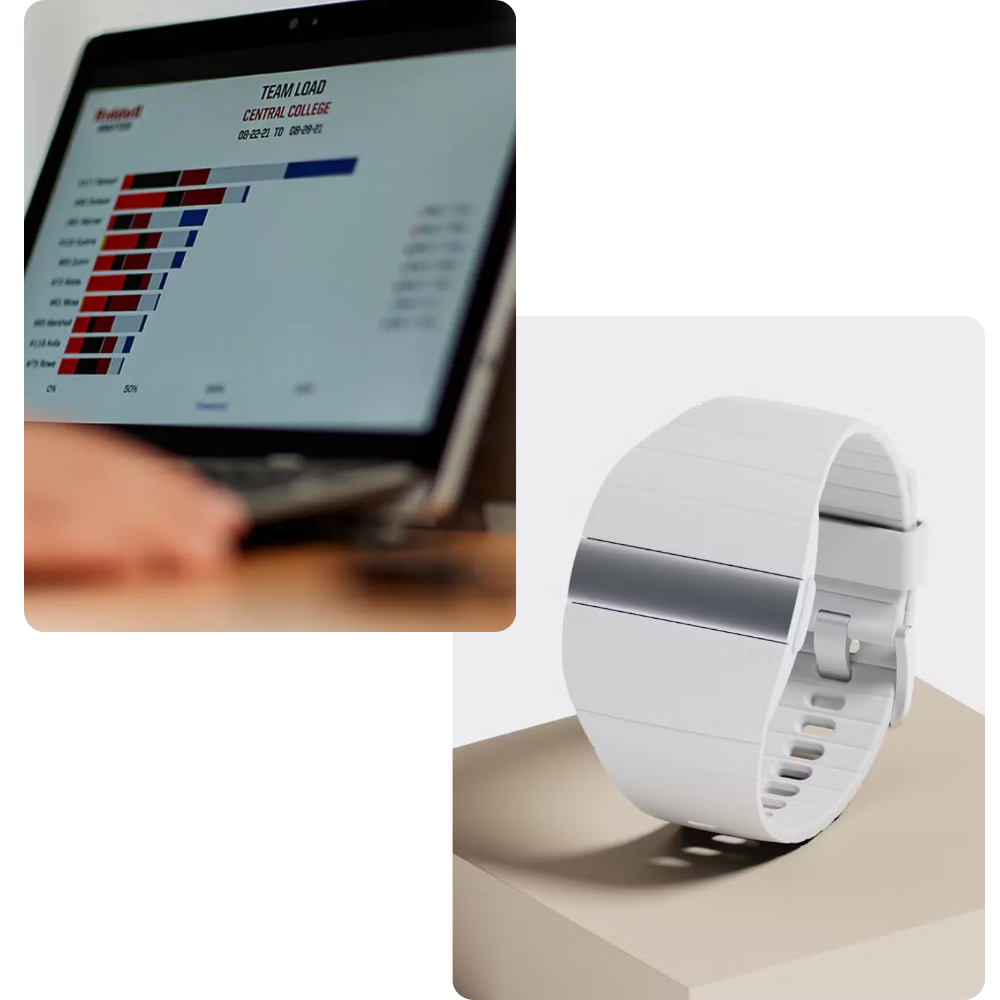
Advantages of AI for HealthTech products
By developing bespoke software and AI models, Simbex leverages multidisciplinary expertise in algorithm development, human factors, and system integration. This approach not only addresses the specific challenges of your medical device but also ensures market readiness and improves adoptability for your product.
Enhanced Predictive Capabilities
AI enables precise predictions and insights, improving patient outcomes through of supervised, unsupervised, semi-supervised, and reinforcement learning techniques
Improved Diagnostic Accuracy
AI technologies such as deep learning enhance diagnostic tools for early disease detection with Deep Learning techniques like CNNs, RNNs, and GANs to aid in early detection of diseases such as cancer.
Personalized Patient Care
AI supports the development of personalized health monitoring systems and tailored health recommendations with real-time data processing.
Operational Efficiency
Automation of routine tasks and data analysis increases efficiency and reduces costs.
Our Approach to AI Solutions
Our team leverages experience in applied research, machine learning, and deep learning techniques for our AI-related projects. Specifically, we have developed significant expertise in areas such as Head Impact Biomechanics, Human Gait, Human Performance, Neuromodulation, Pressure Ulcer Prevention, Fall Prevention, Balance, and Rehabilitation.
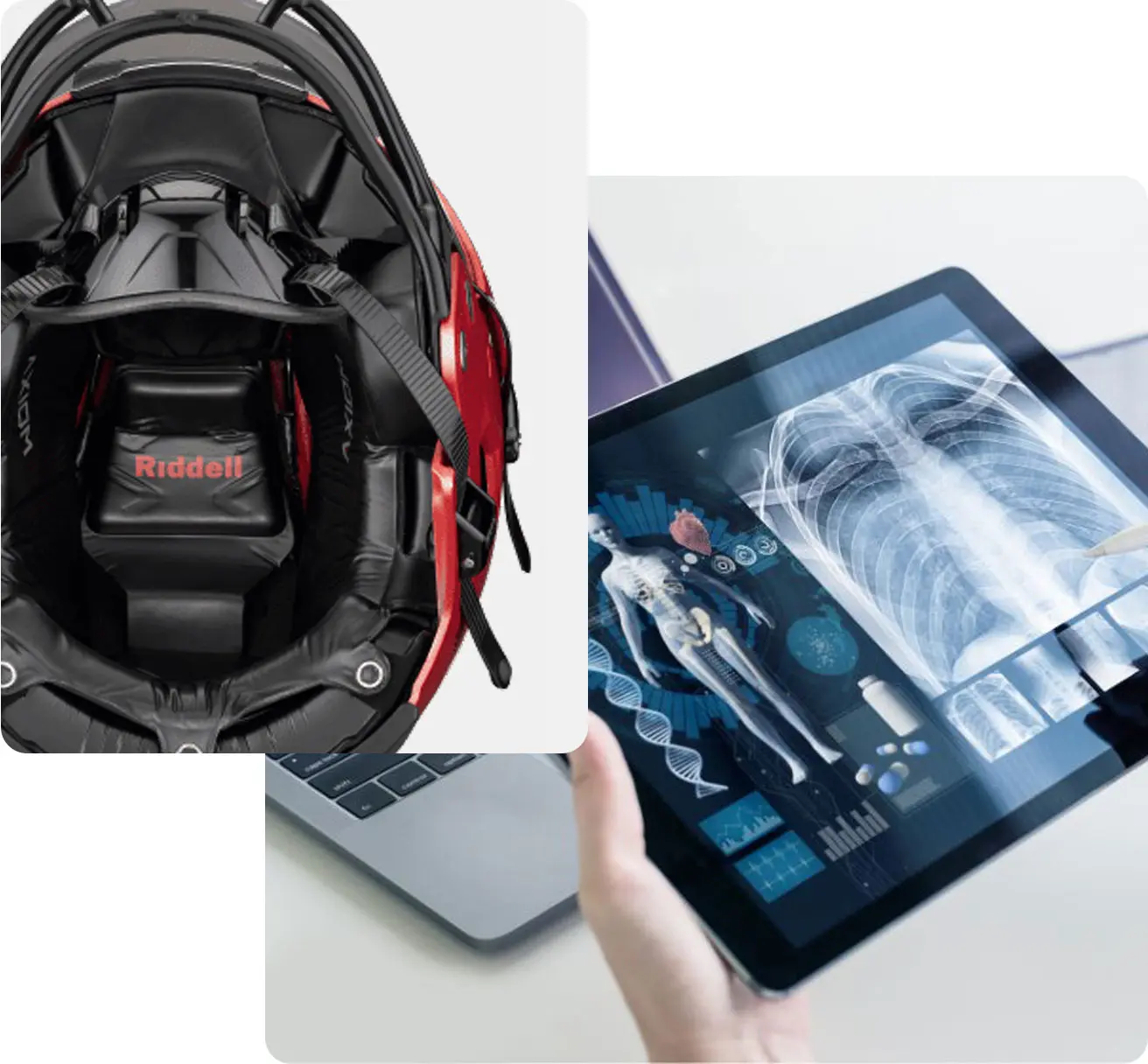
Areas of Applied Research
Basic Research, Translational Research, Data Collection & Analytics, Clinical Study Execution, Experimental Test Design, and Algorithm Development.
Machine Learning (ML) Capabilities
Supervised, unsupervised, semi-supervised, and reinforcement learning techniques empower products to make predictions and gain insights.
Deep Learning (DL) Techniques
Convolutional Neural Networks (CNNs), Recurrent Neural Networks (RNNs), and Generative Adversarial Networks (GANs) transform products by enabling them to develop a perspective and understanding of the world.
Computer Vision Applications
Allows products to “see” and interpret the world through image generation, object recognition, and facial recognition techniques.
How We Work: Collaboration with Customers
Our approach involves a collaborative dialogue with our customers to identify an optimal solution that aligns with their unique needs and limitations. We’re dedicated to crafting a custom AI architecture that is perfectly tailored—neither too complex nor too simplistic—for our customers’ objectives, ensuring they understand the pros and cons of various options.
We enable products to make decisions based on rule-based or physics-based inputs. This field encompasses rule-based systems, physics-based systems, and knowledge engineering applications.
AI Processing Implementation
The world of AI is vast, and where the processing happens can have a big impact on its capabilities.

Edge AI (Sensor/Device)
Edge processing on the sensor puts the processing power and algorithms directly on the device itself. Think of smartwatches with fall detection or cameras with built-in facial recognition.
While this offers benefits like reduced latency (faster response times) and the ability to function in remote locations without an internet connection, it comes with limitations.
Edge devices often have limited computing resources, meaning they can’t handle complex tasks, and have tight power budgets that can affect battery life. Additionally, maintenance and updates can be trickier, and storage space is typically limited.
Edge AI (Mobile)
Running processes through a smartphone or tablet, often as part of an app or service, often as part of an app or service, offers real-time processing with more power than dedicated edge devices.
Mobile AI can also work in remote locations, and updates are generally easier to manage. However, mobile devices still have limited computing resources compared to the cloud, and complex tasks can drain the battery quickly as well as utilize storage space.
Additionally, there can be challenges ensuring the AI works well across different devices and operating systems.
Cloud-based AI
Leveraging the vast computational power and resources of the cloud for AI processing can offer unmatched scalability to handle massive datasets and complex tasks.
The cloud also provides continuous updates and eliminates the need for specialized hardware on-site. Cloud-based AI can handle tasks like generative AI, which requires immense processing power. However, the cloud relies heavily on a stable internet connection, and latency can be an issue.
Using Cloud services can pose additional concerns regarding costs as the workload scales and data privacy as the collection of content grows.